post by Farid Vayani (2020 cohort)
Introduction:
The “Safe and Trustworthy AI” (STAI) summer school, which Imperial College held in July over three days, brought together renowned academics, industry experts and students, providing a platform to learn the cutting-edge research and developments in artificial intelligence (AI). It fostered critical thinking about the ethical issues of AI and its influence on society. The event included discussions on the ethical challenges, advances in Explainable AI (XAI), deep reinforcement learning, AI-driven cybersecurity risks, and the importance of integrating ethical considerations and interdisciplinary collaboration for the development of safe and trustworthy AI systems. The event also provided an opportunity to reflect on the methodologies and constraints of the research paper titled “BabyAI: A Platform to Study the Sample Efficiency of Grounded Language Learning”.
AI’s Ethical Challenges:
The talks and subsequent discussions focused on the effects of AI on job displacement and workers’ rights, where we discussed the delicate balance between artificial intelligence automation and its influence on the work market. Concerns over pervasive surveillance, as well as the necessity for transparent AI governance, were debated. The ethical implications of AI’s inclusion into the legal system were of participants’ interest because of the potential bias and discrimination of AI-driven decision-making.
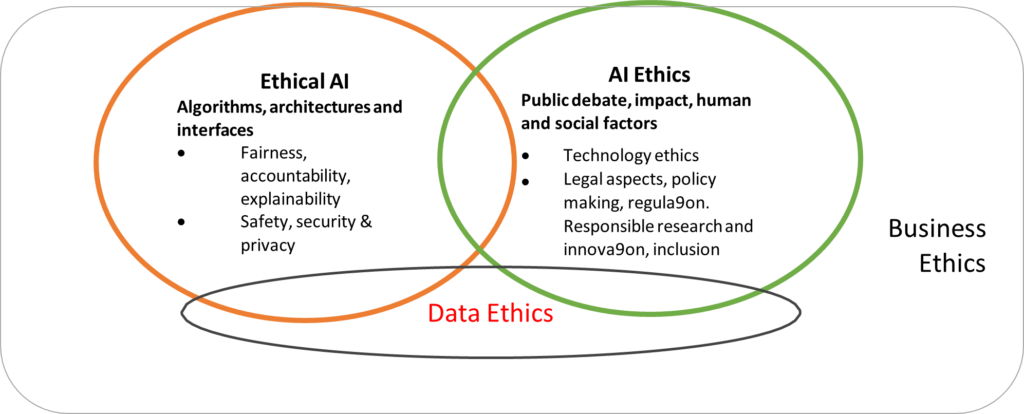
The speaker used real-world examples that raised serious ethical problems, such as the highly criticised algorithm COMPAS (CorrecEonal Offender Management Profiling for AlternaEve SancEons) which is used in the US criminal justice system allegedly perpetuating systemic racial bias. The Cambridge Analytica scandal where data from 87 million Facebook users was exposed via a quiz app, which took advantage of a flaw in Facebook’s API, leading to security and privacy problems which was exacerbated by its use for political purposes. The event surfaced the complexity of the AI landscape and equally the significance of translating legal provisions into efficient business practices.
Explainable AI (XAI):
The event also drew attention to XAI, a key aspect of AI research aimed at improving transparency and interpretability of AI systems. Speakers showed various techniques for interpreting AI models and understanding their decision-making processes, ensuring trustworthiness and accountability in AI applications. XAI has emerged as a significant field of study, addressing the black-box (see Figure 2) nature of complex AI algorithms, and striving to make AI more understandable and explainable to end-users and stakeholders.
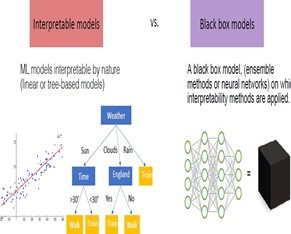
Deep Reinforcement Learning:
Speakers discussed the progressions in deep reinforcement learning (DRL), which has garnered significant attention for its notable capabilities in enabling AI systems to learn complex tasks through trial and error. DRL combines deep learning and reinforcement learning, allowing AI agents to learn from experience and decide in dynamic environments. Discussions revolved around the potential of DRL to revolutionise various domains, such as robotics, gaming and more. However, ethical considerations were highlighted as DRL presents challenges in ensuring safety, fairness, and accountability.
Review of “BabyAI” Research Paper:
The student-led activity involved reviewing the research paper titled “BabyAI: A PlaBorm to Study the Sample Efficiency of Grounded Language Learning.” The paper introduced the BabyAI research platform, designed to facilitate human-AI collaboration in grounded language learning. The platform offered an extensible suite of levels, gradually leading the AI agent to acquire a rich synthetic language, similar to a subset of English. The research presented in the paper highlighted the challenges of training AI agents to comprehend human language instructions effectively. The platform could serve as an important tool for researchers to test methods for DRL, it would suffer from scaling and the opacity of the algorithm which raises the question of whether AI agents learnt the language for human machine interaction or did they just learn to solve the test presented. It calls for further research to improve sample efficiency in grounded language learning to interact with and understand natural language instructions more proficiently.
AI-Driven Cybersecurity:
Speaker from the industry devoted to AI-driven cybersecurity. They showed novel ways that adversaries could exploit AI-generated attacks for automatic discovery of zero-day attacks, methods of malware propagation, and adversarial attacks on defensive systems. The event underscored the necessity for vigilance and collaborative efforts to safeguard digital infrastructures from the evolving AI-based threats. While AI has shown potential to enhance cybersecurity measures, it clearly has introduced new challenges, that demand sophisticated countermeasures.
Ethics and Interdisciplinary Collaboration for Safe AI:
Throughout the event, the importance of integrating ethical considerations into AI development and deployment was emphasised. Ethical AI frameworks are essential to ensure that AI technologies align with societal values and protect individuals’ rights and privacy. Moreover, interdisciplinary collaboration between scientists, engineers, ethicists, policymakers, and other stakeholders is vital for a consistent approach to AI development that prioritises human well-being and safety.
The Way Forward:
The various talks and discussions during the event revealed that regulation and policy alone cannot fully address the complex challenges in managing AI risks such as the European Union’s AI regulation is an important step in the right direction, however, the speakers and participants underscored that more comprehensive approaches are needed to ensure that AI systems function ethically and responsibly. These approaches include integrating solid safety engineering principles into AI development and embedding ethical considerations in the impact assessment, design, validation, and testing.
In conclusion, the STAI event shed light on the ethical challenges and advancements in AI research, emphasising the significance of integrating ethics into AI development, considering distributive fairness, privacy protection, and responsible practices. The quest for ethical AI remains an ongoing journey, where interdisciplinary collaboration, solid safety engineering processes, and thoughtful regulation play pivotal roles in shaping the future of responsible AI technology, one that benefits people.