posted by Gregor Engelmann (2014 cohort)
Emerging economies around the world are often characterised by governments and institutions struggling to keep key demographic data streams up to date. The combination of mass call detail records (CDR) data with machine learning has recently been proposed as a way to obtain this data without the expense required by traditional census and household survey methods. The paper is based on the exploratory analysis of CDR and mobile payment (M-Money) data for Dar Es Salaam, Tanzania, carried out as part of the PhD Research. It forms the basis for a chapter on the potential of mobile phone generated data to supplement traditional surveying methods for socio-economic analysis in urban and peri-urban areas.
The paper was written by N/LAB PhD student Gregor Engelmann in collaboration with the PhD supervisor James Goulding and the N/LAB Data Science Lead Gavin Smith. Moving from an initial analysis of the M-Money data and overcoming both technical and general paper writing hurdles to submitting and ultimately presenting the paper was a prolonged process that took nearly a year.
The major technical hurdle was adding a geographical component to, and cleaning the M-Money data. The dataset is comprised of anonymised logs of mobile payment transactions generated through mobile financial services usage of a Tanzanian Mobile Network Operator. While CDR data has been used in a wide number of areas from epidemiology to mobility and urban analysis with more than 900 papers using CDR data published in the last decade alone, the research on M-Money data is very scarce due to the high difficulty of obtaining such data sets from Mobile Network providers. Organisational hurdles included identifying an appropriate journal or conference venue, making sure that both abstract and paper are ready by the relevant deadlines, and raising enough funding for conference travel. The submission to the initially identified conference was ultimately delayed as the paper required more work before submission to IEEE Big Data in early summer 2018. Being based in the same lab made collaborating on the paper easier as we could have regular in-person meetings to review the paper. In addition to in-person meetings, we extensively used Overleaf, an online LaTeX platform, which allows for both collaborative writing and editing. LaTeX has the advantage of being able to handle equations, figures, indices etc. better than traditional writing software such as Word. LaTex (and by extension BibTex) also offers more effective bibliography and reference management while allowing for changes in formatting and style with a few simple lines of code.
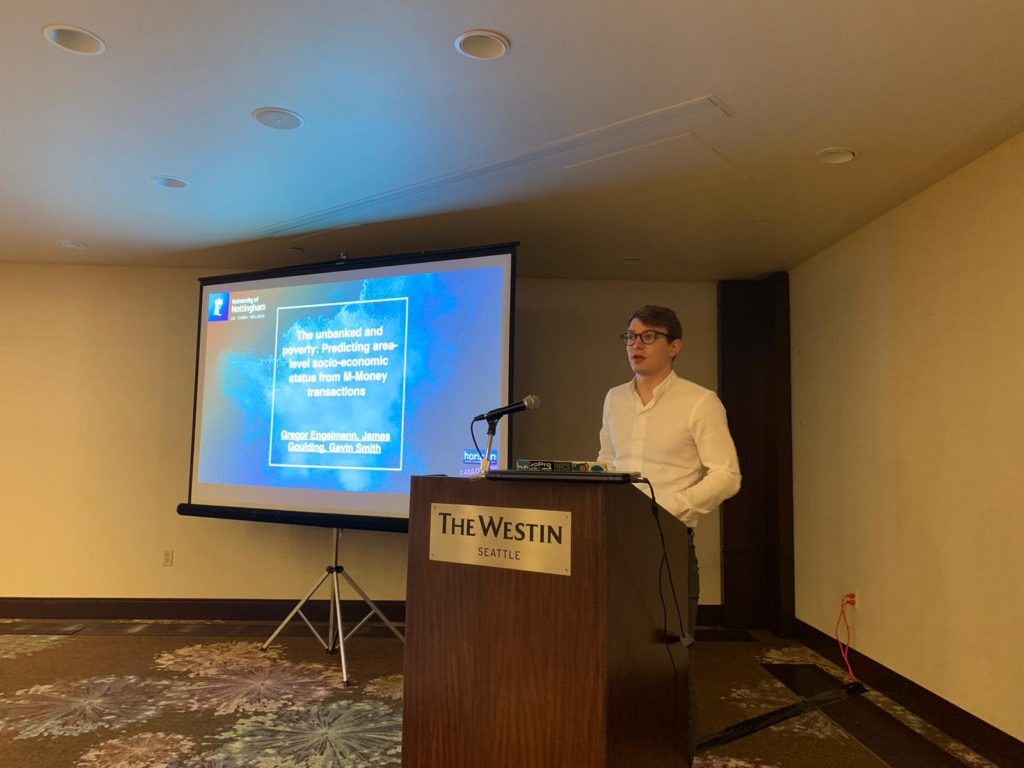
The reviewers’ response to the paper was positive and it was ultimately accepted as one of 98 regular papers (acceptance rate 18.9%) without the need for major changes. In addition to funding from the Business School and CDT, the paper was successful in securing one of the limited Student Travel Awards offered by the conference organisers to cover travel and registration costs to Seattle. The paper was presented to a mixture of academic and practitioner audiences as part of the Big Data Applications: Society track at the IEEE Big Data 2018 conference in Seattle, WS in December 2018.
N/LAB PhD student Gregor Engelmann’s paper: The Unbanked and Poverty: Predicting area-level socio-economic vulnerability from M-Money transactions, accepted and presented as a regular paper into the IEEE Big Data conference 2018 is available online via the publisher (paywall) https://ieeexplore.ieee.org/abstract/document/8622268/figures#figures and Nottingham University eprints http://eprints.nottingham.ac.uk/55720/