post by Gift Odoh (2022 cohort)
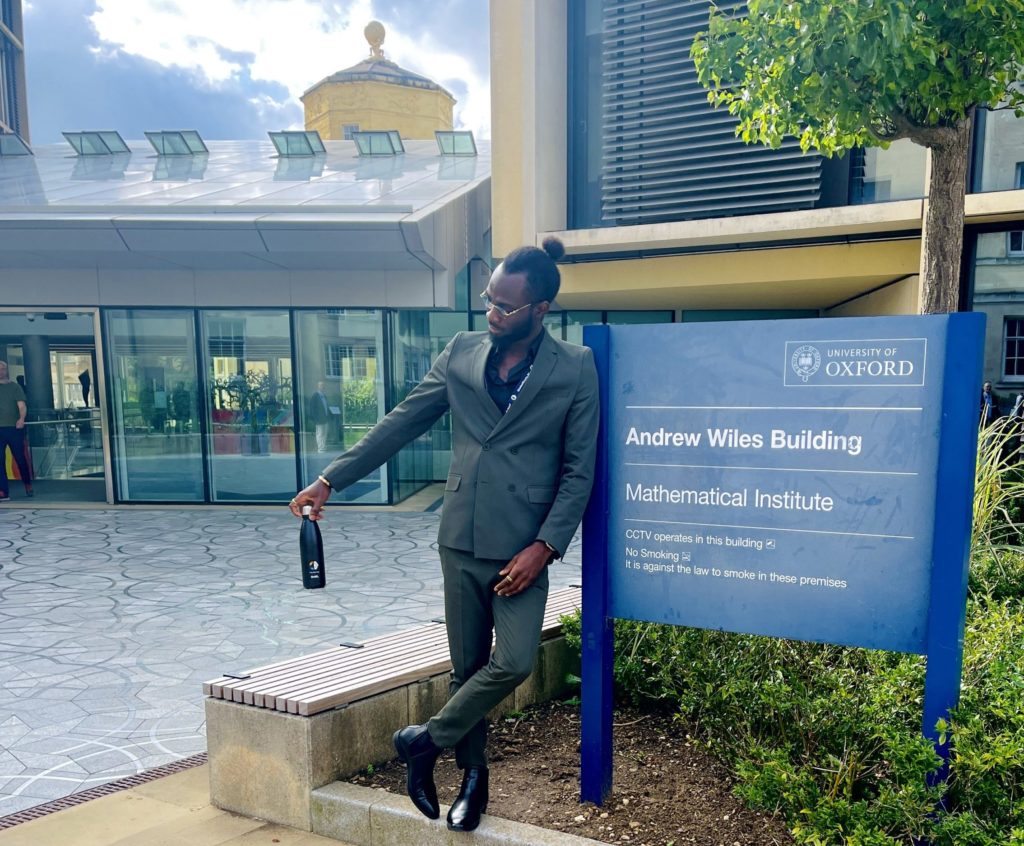
Between the 13th and 16th of July, 2023, I attended the Oxford Machine Learning Summer School at the Mathematical Institute of the University of Oxford for health applications. The course organised by the AI for Global Goals in partnership with the University of Oxford’s Deep Medicine and CIFAR, was focused on advanced areas in machine learning (ML), ranging from statistical and probabilistic approaches to representation learning (an ML approach based on representations of data that make it easier to extract useful information when building classifiers or other predictors [1]), specialised techniques for complex data structures, computer vision, knowledge representation and reasoning, and the integration of symbolic and neural approaches for enhanced AI capabilities.
My interest in the school was from the opportunity for exposure to valuable exploration into ML’s diverse applications and expectations towards uncovering connections between ML techniques and their relevance to my PhD research, which focuses on robotic teleoperation and human-robot interaction, particularly concerning mental workload indicators and how they can inform robotic assistance schemes in teleoperation. I also saw it as an opportunity to meet people of similar interest in the field and visit the renowned city of Oxford and its University of Oxford colleges, some known to have rich histories.
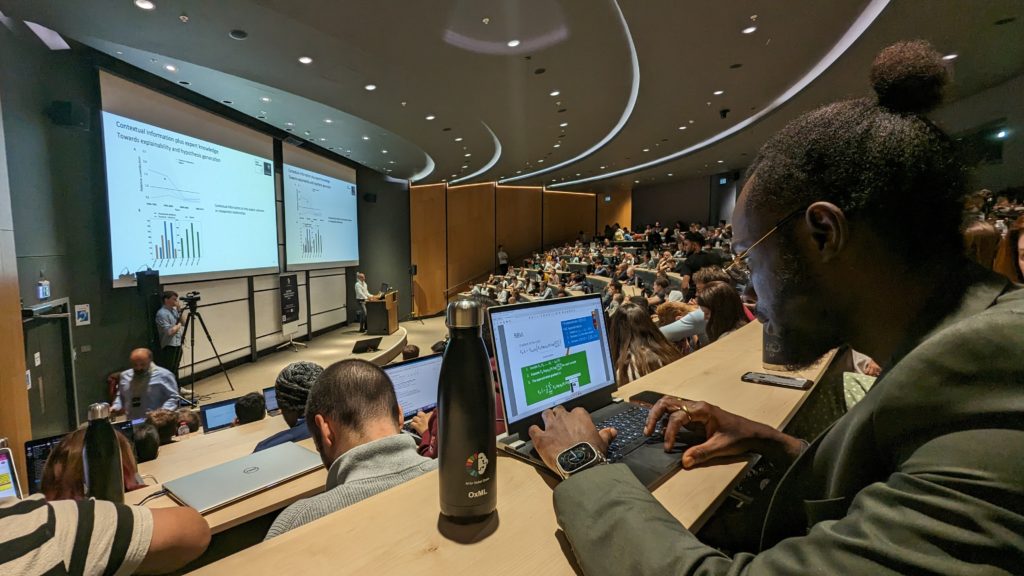
The first couple of sessions focused on how we understand our environment as humans – covering how we represent the world and its actual truth from different observations. These sessions paved the way for representation learning and how intelligent systems can extract useful information from features present in data, particularly when there are no labels. S. M. Ali Eslami, in his session on representation learning without labels, underscored the importance of labels to effective machine learning but demonstrated how learning can still be achieved when label collections are impossible by showing how different encoders make representations (understanding) of data from inputs as well as how this is reversed though generative models that make real-world estimates of this representations. While most sessions focused on probabilistic models based on generative techniques and casual machine learning, which focus on the learning process, Professor Pietro Lio from Cambridge presented an intriguing session on graph representation learning, which is a form of machine learning useful for organised data in the form of networks or graphs where points of data (nodes) are connected with edges (relationships), making an interesting case for utilising graphs as they are everywhere in research. Although most of the application areas were in molecule generation for proteins and drugs, its application in extracting meaningful insights and predictions from relational data can be applied to model robotic assistance schemes that respond to mental workload within a complex framework where nodes can represent operators’ mental states such as attention levels, stress levels or task demands and the edges can signify the relationship and dependencies between them.
Another key aspect of the course was computer vision for ML. Some of these sessions were on the evolution of computer vision and its techniques and unsupervised visual learning for ML applications, particularly medical imaging. Understanding the progress of computer vision and where it stands today has practical implications for my work, given that vision is integral to teleoperation interaction. Christian Rupprecht presented the stages for understanding a scene, including scene classification, where the general scene is described; object detection, in which various objects in the scene are identified; segmentation, which involves dividing the scene into meaningful, distinct parts and regions; scene graph, which describes the positional relationship between objects; description in which an improved interpretation of the scene is obtained and hierarchy which informs the how scenes are decomposed into objects, parts and materials.
It was, however, useful that the summer school was not just about machine learning techniques in isolation. The segment on Bridging Machine Learning and Collaborative Action Research emphasised the importance of collaboration, especially in areas like digital mental health. For example, the limitations of applying findings from social media data for health states generalisation, methodological issues, challenges understanding other attributes (e.g. offline attributes) and threats of relying on single data sources. This challenge emphasises the indispensability of interdisciplinary collaboration, which resonated deeply with my belief in merging human-robotic interactions with other disciplines for a more holistic approach to tackling the interdepending challenges of robotic assistance in teleoperation. Although it seemed to me that some of the techniques were unique in their approach and application to specific conditions, I see an opportunity for careful examination into how some approaches could come together to enhance robotic autonomy and facilitate better human-robot interaction.
In conclusion, the school has added depth to my understanding by expanding my academic horizon to approach my research through the sessions, including those that felt directly applicable and the seemingly marginally relevant ones. It is also noteworthy that the school was also an opportunity to meet other PhD students from diverse backgrounds and corners of the world. Our interactions provided valuable global perspectives on the various ML applications in health research. I must also add that I had the opportunity to explore the historic city of Oxford and its renowned University of
Oxford colleges both through guided tours and lone walks, which offered a cultural immersion and ignited a sense of academic inspiration. My interactions with students, researchers and industry professionals allowed me to forge meaningful connections in machine learning that broadened my understanding and opened doors to potential future collaborations and opportunities. Overall, it was a transformative learning experience that equipped me with a global network to renew my sense of purpose in my research and professional journey.
[1] Y. Bengio, A. Courville, and P. Vincent, “Representation learning: A review and new perspectives,” IEEE Trans Pattern Anal Mach Intell, vol. 35, no. 8, pp. 1798–1828, 2013, doi: 10.1109/TPAMI.2013.50.