post by Ana Rita Pena (2019 cohort)
Interning at Capital One
Between May and October 2021 I held a part-time internship with my Industry Partner, Capital One. Capital One is a credit card company that launched its operations in the UK in 1996 and has its parent company in the US. The company is known for being technology driven and in the specific UK case focusing on credit building cards as their main product.
My internship with Capital One UK consisted of working on several projects as part of their Responsible AI initiative due to my interest in topics related to “ethical” machine learning and FACct/FATE (Fairness, Accountability, Transparency and Explainability).
The Responsible AI initiative initially consisted of three projects: the Trustworthy Algorithm Checklist, Global Methods and Individual Level Methods. The Trustworthy Algorithm Checklist project was already under way when I joined the company in May 2021. The project consisted of creating a checklist for Model Developers to complete during the model development process, in order to instigate some reflection and mitigation of ethical risks and consequences associated with the new model. The Global Methods project was subdivided in two parts. Generally, the project aimed to evaluate different explainability methods and provide some guidance and recommendation on different methods to be adopted internally by the Data Science team. The first part consisted in interviewing stakeholders from different departments to have a better understanding about which information each of them needed about the model and the second part consisted of a technical evaluation of the tools. Finally, the third project, Individual Level Methods, aimed to explore how consumers understand different explainability methods to represent their individual outcome. This third project never went ahead due to lack of time.
On my day to day, I worked within the Data Science Acquisition team as my manager was based on this team and he spent a percentage of his time working on the Responsible AI Initiative, however my workflow was separate to the rest of the team. Being able to attend the team’s meetings allowed me to have a better understanding of the workings of the company and the processes involved in model development and monitoring.
In the following sections I will describe in more detail the two projects I worked on as well some general reflections and thoughts on the internship.
Trustworthy Algorithms Checklist
Over the last few years there have been several stories in the press of algorithms which are implemented and end up having unintended consequences which negatively affect users, for example the UK’s A-level grading algorithm controversy, which ended up affecting students from lower socio-economic backgrounds more negatively than other students by deflating their grades the most. This has led to research in the areas of “ethical” AI to cross into real world applications. The Trustworthy Algorithm Checklist Project aims to design a checklist which will make model developers actively reflect on unwanted impacts of the model they are building as part of the development process. After the checklist completion it would then go through an ethics panel composed of stakeholders from different business departments within the company, for an approval process. The initial draft checklist was divided into three sections: Technical Robustness, Diversity Non-discrimination and Fairness and, finally Accountability.
The next iteration of the checklist design created more sections which were based on the Ethical Principles for Advanced analytics and Artificial Intelligence in Financial Services created by UK Finance, which is a trade association for the UK banking and Financial Sector. Ending up with the following five sections: Explainability and Transparency, Integrity, Fairness and Alignment to Human Rights, Contestability and Human Empowerment and Responsibility and Accountability. It was at this stage that I joined the company and started working on this project. The project involved stakeholders from the legal and compliance department as well as from the data science department. This second iteration of the checklist was trialed with a model that was in the initial stages of development, from this trial it was noticed that most of the answers to the prompts in the checklist were mentioned to already be covered by existing Capital One Model Policy. In order to avoid the Checklist becoming just another form that needs to be submitted, the team decided to put more emphasis on the ethics panel discussion meeting and have the checklist being an initial prompt in the discussion in order to foster critical reflection which is aided by having stakeholders that come from different backgrounds and hence bring different perspectives.
While this project initially only focused on the algorithmic aspect of decision making, the team involved discussed the possibility of expanding the checklist to the process of development of Credit Policies. It is the combination of the Algorithmic Risk Assessment with the Credit Policy which will end up impacting the consumer and hence the need to critically evaluate both these parts.
Explainability Toolbox
Global methods are a set of tools and visualisations to help better understand the way complex machine learning models work at an overall, rather than individual level. These methods can focus on the variables, for example which variables have bigger impact on the result or how different variables are related among themselves, or the general decision rules of the model, for example summarise a complex method by approximating it to a set of simple decision rules.
Including explainability methods on the machine learning work process is quite important as these allow one to verify the behaviour of our model at any time and check if it is working well and as expected.
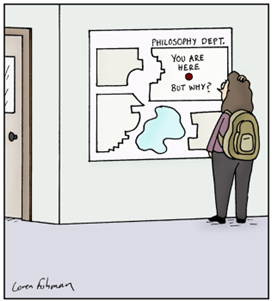
When this project was first discussed with me it consisted of implementing and comparing different explainability methods and packages implementations (both developed internally as well as open-source tools) in order to propose a set of tools to uniformise the tools used by different teams within the Data Science Department. Due to my interdisciplinary experience in Horizon and partly working within the Human Factors field, I was aware that the technical components of the explainability methods are not the only factor that affect how well technology is adopted and used in an institutional setting. In order to address the social aspect of this task I suggested having a series of interviews with different Stakeholders that interact with the Data Science team, in order to have a better understanding of what information they needed on the models and would like to better understand and on their views on the methods that were currently implemented. Doing these interviews allowed me to understand better what different roles and departments did and how they interacted, as previously I had mainly only interacted with the Data Science department.
From the interviews I learned that stakeholders from more business-related roles as well as higher level roles were interested in being able to translate how changes in the model’s behaviour impact business, e.g. in terms of number of people given loans or in terms of profit or number of defaults. Stakeholders from the technical departments were also aware of the shortcomings of the methods they currently adopted but had not had the time to test alternatives in their workdays. From the interviews I created a list of guidance when presenting technical materials to stakeholders as well as identified several fields to evaluate on the second part of the project.
In the second part of the project, I compiled different packages/libraries (open source and internal packages developed by different C.O departments and countries) to test their methods and give guidance on what could be beneficial to implement across the Data Science Department. During this process I learned that different branches of C.O. in different countries use different coding languages as well as that different models from different Data Science teams had different characteristics due to their different needs and what historically their department and branch have implemented. This meant that oftentimes different teams had to create their packages from scratch to meet the specificities of their model, even if they were using the same tools which could have been avoided if there was uniformisation of the language or model construction approach.
Final Reflections
This was my first time working in Industry and I was very pleasantly surprised with the importance that Capital One puts in research, running internal conferences and having specialised research centres (in the US, which is their biggest market). This was further encouraged by the very open and collaborative work environment, for example the Responsible AI UK initiative I was involved with had regular meetings with other research teams within C.O. working in the same field.
While the company had very good intentions and initiates in different projects just like the ones I worked in, the reality of the scale of the UK branch meant that all of the team (apart from myself) only worked on the Responsible AI initiative 10% of their time on top of their team ’s roles. The Explainability Toolbox project also showcased the drive to optimise processes across departments, even if hard to accomplish at scale due to logistical constraints.
Overall, my internship at Capital One gave me a better understanding of the Consumer Credit Industry and the way different departments come together to be able to provide a financial product to the consumer.