post by Alexa Velvet Spors (2017 cohort)
Hi everyone,
I’m currently recruiting for my last PhD study, a research game jam!
Please see details below – maybe it might be interesting for you, or you know someone who might enjoy it?
If you have Twitter, I’d massively appreciate a retweet or like to gain some visibility in the algorithmic soup 😊!
https://twitter.com/caringsystems/status/1412053592106799107
Thank you!!
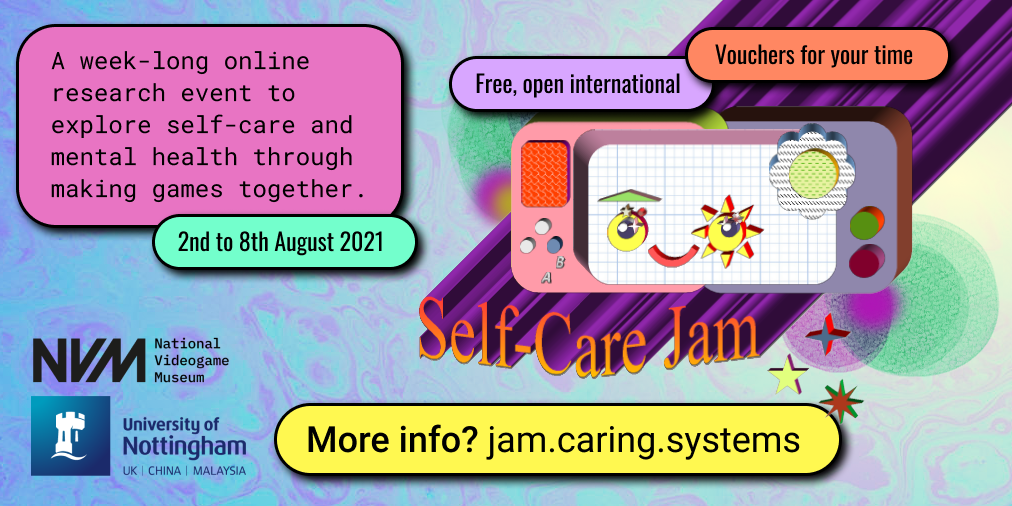
Self-Care Jam
-
- A week-long research game jam
- 2nd to 8th August 2021
- Let’s think, make and research games, mental health and caring technologies together.
- £100 in Amazon vouchers + 2 tickets to the NVM for your time!
Register your interest here:
https://forms.office.com/r/nSNz7wx6FB
Huh? What’s this project all about?
-
- Do you have interest in mental health and/or self-care?
- Are you someone who enjoys video games?
- Do you think games can be more than just entertainment?
- Do you enjoy making and designing things with other people?
Infomercial introduction aside: Hi, we are Velvet Spors and Imogen Kaufman, PhD students at the University of Nottingham. Together with the National Videogame, we are researching how mental health, self-care, and games can come together to create new, exciting, and meaningful technology.
Games connect us, make us cry or laugh (or both at the same time!). They also allow us an open, safe space to explore feelings and make sense of ourselves. For this very reason, Velvet brought people together to create a community resource that other people can use as a starting point for designing and making self-care tech that is open-minded, genuine, and maybe different in its approach: The Caring Systems toolkit.
We are interested in testing out this toolkit with critical, curious people who enjoy gaming and who think technology can support our everyday lives and mental health. Does it work at all? What needs to be improved? Which changes should we make?
What would I have to do?
Monday-Thursday: Explore the toolkit in our own time + fill out a survey. (You can join us for optional 1h activities!)
Friday: Join us for a 2h kick-off event.
Saturday-Sunday: Spend 4h each day making and thinking with others.
Afterwards: Fill out a survey + an interview about your jam experience.
Receive a £100 Amazon shopping voucher for your time (or the equivalent in your country’s currency) + 2 tickets to the NVM!
Who are we looking for?
– Game jamming/making things collaboratively with other people is your jam (literally or figuratively).
– Exploring the potential of games and self-care technologies sounds fun to you.
– You enjoy making games and/or technology (or you have thought about making or designing it!).
– You have somebody local to you, who could check in on you during the game jam.
– You are 18+ years old and you are currently not experiencing a mental health crisis.
– You can commit to the game jam times (~10h over the week 2nd to 8th August 2021, especially 6-8th August 2021).
You do not have to be able to code or design — everybody is welcome, regardless of skill level.
The jam is open internationally.
Sounds cool! Sign me up!
Register your interest here:
https://forms.office.com/r/nSNz7wx6FB
All the best,
velvet
/